Assessment of Accelerated Stress Testing Data Using Tensor Decomposition Methods
In this DuraMAT project, we seek to develop machine learning (ML) tools for decompositional analysis of photovoltaic (PV) image sequences to identify constituent spatial structures that may have separate impacts on current-voltage (IV) metrics.
These new tools will separately display the evolution of distinct spatial patterns in PV image series at different rates upon degradation, enabling us to extract separate degradation rates and assess their impacts on power loss.
The PV industry is simultaneously targeting long warranties (>50 years) and new materials/designs for high-energy-yield modules, requiring an advanced methodology to forecast long-term durability of products with un-proven materials combinations. This work seeks to develop a pathway towards faster learning cycles in accelerated stress testing by combining multi-modal data from sequential stress tests with data-driven ML tools.
Extended, sequential, and combined stress testing methods are gaining popularity for assessing durability of PV modules/materials beyond the early-stage mortalities identified in the standard tests in IEC 61215. Importantly, multiple degradation mechanisms can proceed simultaneously, and their separate contributions to the overall power loss should ideally be quantified. We traditionally use different measurement types to qualitatively assess the possible simultaneous degradation mechanisms.
Core Objective
Location
National Renewable Energy Laboratory
Applications
The tools developed here are generally applicable to sequential image analysis of PV module or other material degradations to identify key degradation modes and quantify their impact to relevant performance characteristics. Further, these capabilities can be used to model the degradation of the modules forward in time by following the trajectory of key modes.
Availability
The developed tools will be open-sourced and made publicly available on GitHub.
Contact
To learn more about this project, contact Andrew Glaws.
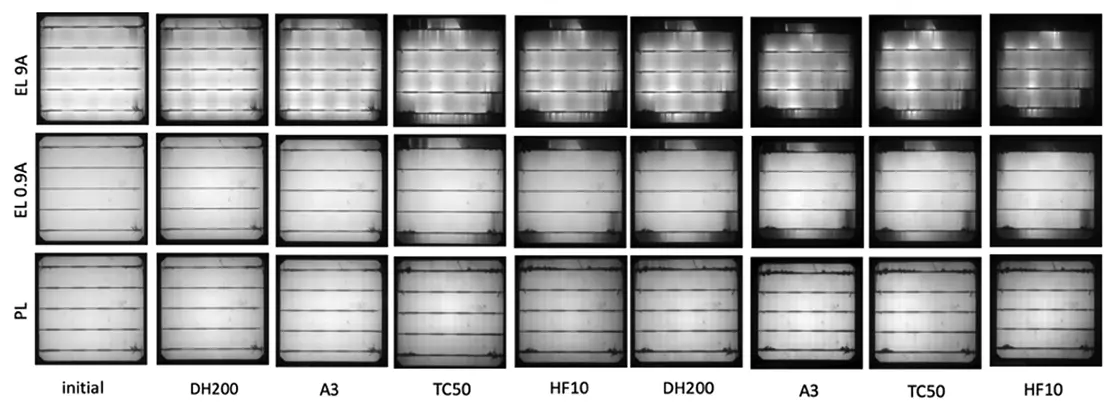